(Edit: 2024-06-21)
Presentation (in Chinese or English)
- 厘米级分辨率无人机定量遥感植被生理变量估算 (北京市农林科学院,受邀报告) [PDF]
- Retrieving understory gap fraction using an energy dimidiate model with airborne waveform LiDAR (SilviLaser, 2023, London) [PDF] [Video]
- 基于多源遥感与生长模型的火后森林受损评估与恢复预测 (第一届多源遥感技术及应用学术论坛,北京) [PDF]
Ultra-high-resolution retrieval of vegetation variables (traits)
To better monitor canopy structure and function at very fine scale, we develop algorithms to estimate fractional cover (fCover), leaf area index (LAI), fractional intercepted photosynthesis active radiation (fIPAR), and advanced biochemical traits such as water content and leaf chlorophyll content (LCC), using a wide range of sensors (RGB, multispectral/hyperspectral camera, LiDAR, thermal camera, etc.).
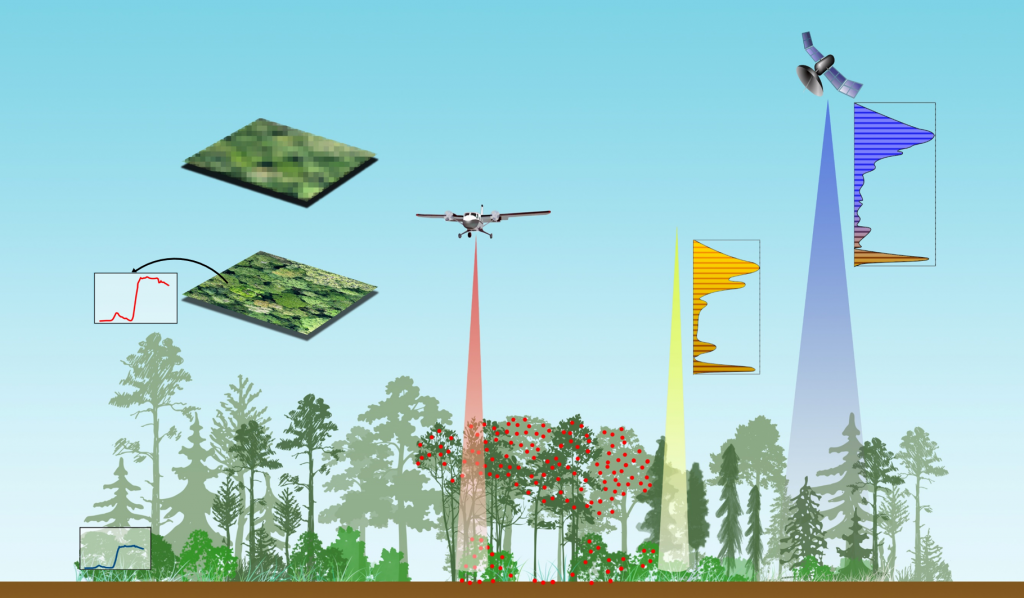
- Li, L.*, Mu, X., Jiang, H., Chianucci, F., Hu, R., Song, W., Qi, J., Liu, S., Zhou, J., Chen, L., Huang, H., Yan, G., 2023. Review of ground and aerial methods for vegetation cover fraction (fCover) and related quantities estimation: definitions, advances, challenges, and future perspectives. ISPRS J. Photogramm. Remote Sens. [HTML]
- Li, L.*, Mu, X., Chianucci F., Qi, J., Jiang, J., Zhou, J., Chen, L., Huang, H., Yan, G., Liu, S., 2022. Ultrahigh-resolution boreal forest canopy mapping: combining UAV imagery and photogrammetric point clouds in a deep-learning-based approach. International Journal of Applied Earth Observation and Geoinformation. [HTML]
- Li, X., Li, L.*, Ni, W., Mu, X., Wu, X., Vaglio Laurin, G., Vangi, E., Sterenczak, K., Chirici, G., Yu, S., Huang, H., Qi, J.*, Validating GEDI tree canopy cover product across forest biomes using co-registered aerial LiDAR data. ISPRS J. Photogramm. Remote Sens. [HTML] [Corrigendum]
- Li, L., Mu, X.*, Macfarlane, C., Song, W., Chen, J., Yan, K., Yan, G., 2018. A half-Gaussian fitting method for estimating fractional vegetation cover of corn crops using unmanned aerial vehicle images. Agric. For. Meteorol. 262, 379–390. [HTML]
- Yan, G., Li, L.*, Coy, A., Mu, X., Chen, S., Xie, D., Zhang, W., Shen, Q., Zhou, H., 2019. Improving the estimation of fractional vegetation cover from UAV RGB imagery by colour unmixing. ISPRS J. Photogramm. Remote Sens. 158, 23–34. [HTML]
- Li, L., Chen, J., Mu*, X., Li, W., Yan, G., Xie, D., Zhang, W., 2020. Quantifying understory and overstory vegetation cover using UAV-based RGB imagery in forest plantation. Remote Sens. 2020, Vol. 12, Page 298 12, 298. [HTML]
- S Cai, W Zhang, S Jin, J Shao, L Li, S Yu, G Yan, 2021. Improving the estimation of canopy cover from UAV-LiDAR data using a pit-free CHM-based method. International Journal of Digital Earth. Page 1-16. [HTML]
- Qi, J., Xie, D.*, Li, L., Zhang, W., Mu, X., Yan, G., 2019. Estimating Leaf Angle Distribution From Smartphone Photographs. IEEE Geosci. Remote Sens. Lett. 16, 1190–1194. [HTML]
High-quality radiometric and geometric correction for multi-modal data (image and LiDAR)
The prerequisite of vegetation variable retrieval and further applications is high-quality analysis-ready data. We proposed a range of methods to radiometrically and geometrically correct the raw UAV data to highly reliable reflectance factor or point cloud or waveform, where the noise, vignetting effect, atmospheric effect, BRDF effect, incompleteness and occlusion effect were considered.
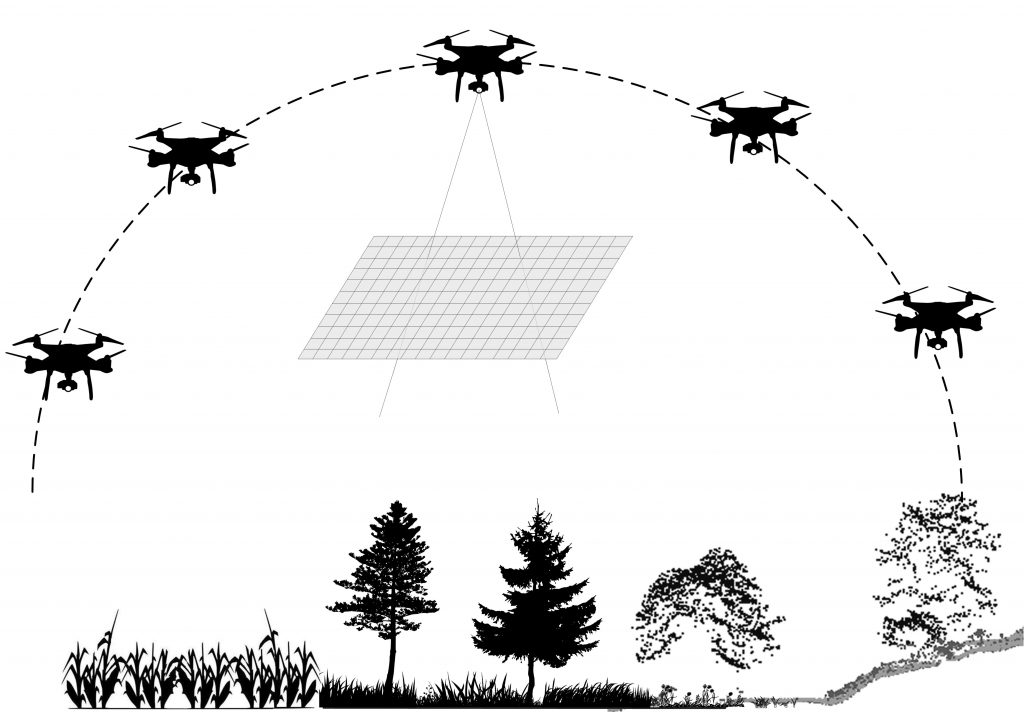
- Li, L., Mu, X.*, Qi, J., Pisek, J., Roosjen, P., Yan, G., Huang, H., Liu, S., Baret, F., 2021. Characterizing soil background BRDF in open forests using UAV-based multi-angular images. ISPRS J. Photogramm. Remote Sens. [HTML]
- Li, L., Mu, X.*, Soma, M., Wan, P., Qi, J., Hu, R., Tong, Y., Zhang, W., Yan, G., 2020. An iterative-mode scan design of terrestrial laser scanning in forests for minimising occlusion effect. IEEE Trans. Geosci. Remote Sens. [HTML]
- Zhang, W.*, Zhao, J., Chen, M., Chen, Y., Yan, K., Li, L., Qi, J., Wang, X., Luo, J., Chu, Q., 2015. Registration of optical imagery and LiDAR data using an inherent geometrical constraint. Opt. Express 23, 7694. [HTML]
Multi-scale vegetation growth and disturbance assessment
The synergies of UAV and satellites offer a huge opportunity for vegetation growth and disturbance (e.g., disease, fire) monitoring at a wide range of scales. We leverage the “ground truth” provided by UAV observations to calibrate and validate satellite-based outcomes.
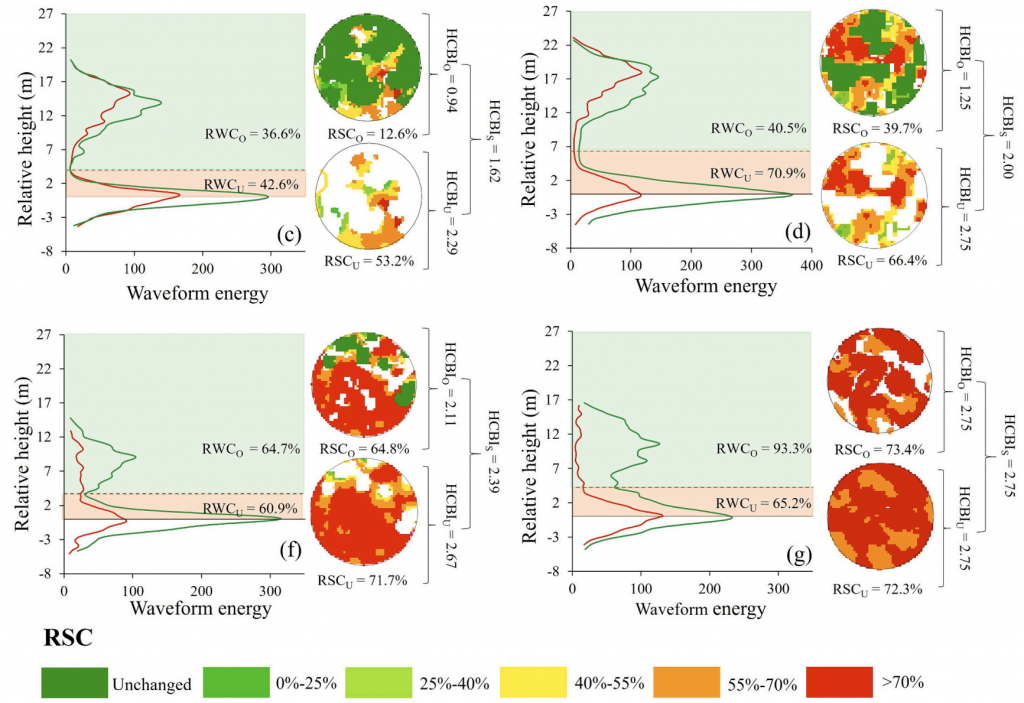
- Lin, S., Li, L.*, Liu, S., Gao, G., Zhao X., Chen, L., Qi, J., Shen Q., Huang, H. 2024. Stratified burn severity assessment by integrating spaceborne spectral and waveform attributes in Great Xing’an Mountain. Remote Sensing of Environment [HTML]
- Lin, S., He, Z., Huang, H., Chen, L., Li, L.*, 2022. Mixed forest-specific calibration of the 3-PGmix model parameters from site observations to predict post-fire forest regrowth. Forest Ecology and Management. [HTML]
- Lin, S.’, Li, L.‘, Liu, S., Yang, S., Lin, D., Zhao, X., Chen, L., Huang, H.*, 2024. Predicting post-fire forest recovery of a high-severity burned area using a process-based 3-PG model with bi-temporal Landsat imagery in Great Xing’an Mountain. Forest Ecology and Management. [HTML]
- 童彤,李林源*,罗涛,黄华国,联合微波与光学时间序列数据的马尾松林松材线虫病遥感识别,北京林业大学大学学报